The Power of Malware Machine Learning in IT Services
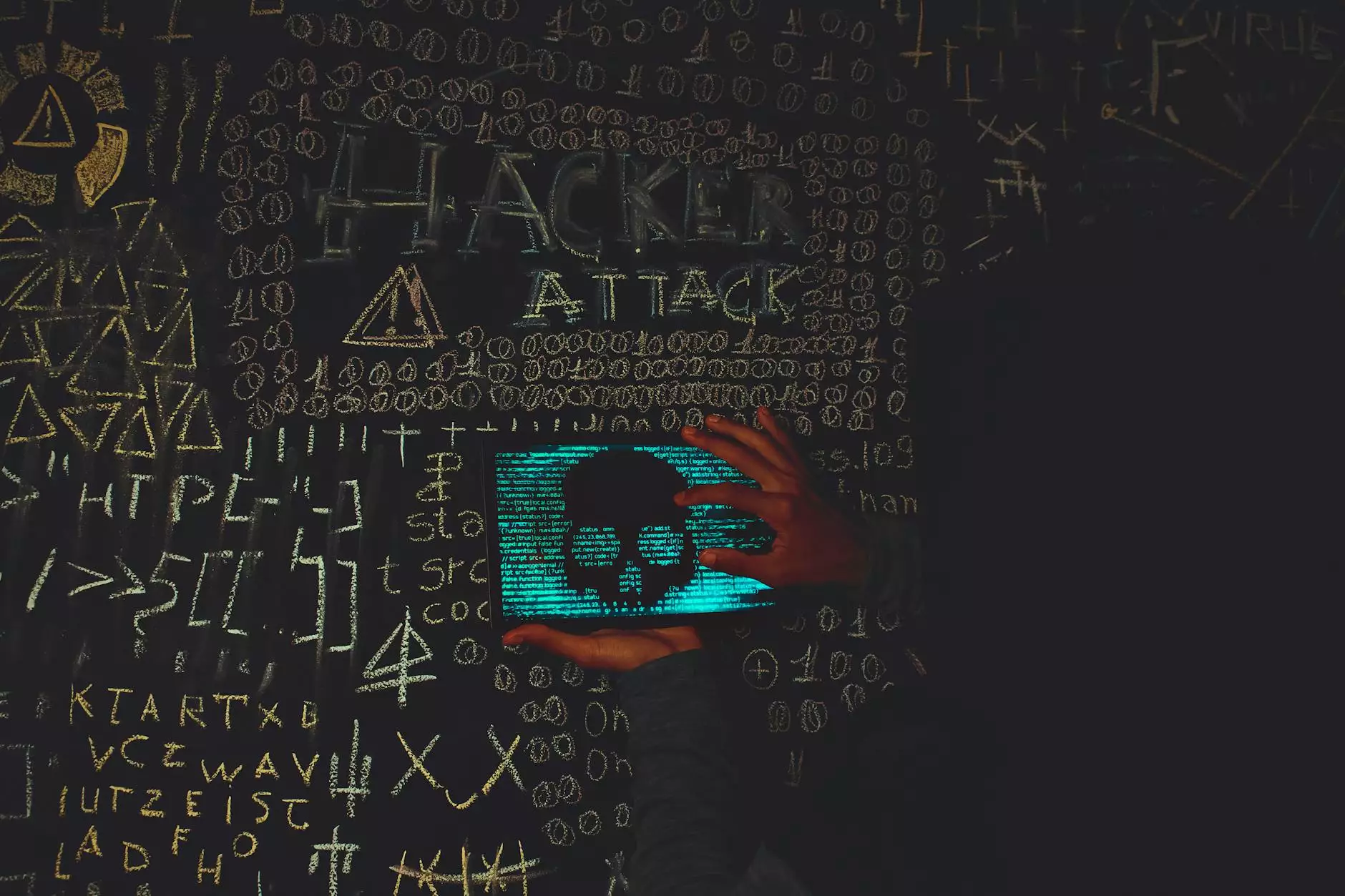
In today's ever-evolving digital landscape, where cyber threats loom large and data breaches make headlines, the implementation of malware machine learning represents a transformative approach to cybersecurity. As businesses increasingly rely on technology, the need for robust IT services and security systems has never been more critical.
Understanding Malware and Machine Learning
Before diving into the specifics, it's essential to grasp what malware and machine learning entail:
- Malware: Malicious software designed to harm, exploit, or otherwise compromise computers, networks, or devices.
- Machine Learning: A subset of artificial intelligence that enables systems to learn and improve from experience without being explicitly programmed.
The Intersection of Malware and Machine Learning
The convergence of malware and machine learning is a game-changer for IT services. As cybercriminals develop increasingly sophisticated methods to write malware, traditional security systems struggle to keep pace. This is where machine learning, with its ability to analyze vast amounts of data and recognize patterns, comes into play.
How Malware Machine Learning Works
Malware machine learning utilizes algorithms that dig through millions of data points to identify behaviors associated with malware. Here’s a breakdown of the process:
- Data Collection: Software samples, historical data from previous threats, and system behaviors are gathered.
- Feature Extraction: Significant characteristics of the data are identified, such as code behavior or network traffic patterns.
- Model Training: Machine learning algorithms are trained using labeled datasets (classified as malware or benign). Common algorithms include decision trees, neural networks, and deep learning models.
- Detection and Response: The trained model is deployed to monitor systems in real-time, flagging potential malware threats based on learned patterns.
The Benefits of Malware Machine Learning in IT Services
Implementing malware machine learning offers numerous advantages for businesses, particularly in enhancing IT services and security systems:
1. Enhanced Threat Detection
Machine learning models can identify new and evolving threats that traditional signature-based detection methods may overlook. By analyzing typical user behaviors and system functions, these models can detect anomalies that signify a malware outbreak.
2. Automation of Security Processes
With the ability to function autonomously, machine learning-driven tools significantly reduce the time IT professionals spend on manual threat assessments, allowing them to focus on strategic initiatives.
3. Improved Accuracy
False positives have been a significant hurdle in cybersecurity. However, machine learning improves accuracy by distinguishing legitimate anomalies from genuine threats, ensuring that fewer business operations are disrupted unnecessarily.
4. Predictive Analysis
Machine learning not only helps in identifying existing threats but also in anticipating future attacks. Utilizing historical data, businesses can plan and bolster defenses proactively.
Case Studies: Successful Implementation of Malware Machine Learning
Several businesses have successfully integrated malware machine learning into their security frameworks. Here are two notable examples:
Case Study 1: Fortune 500 Company
A Fortune 500 company facing frequent data breaches implemented a comprehensive machine learning security solution. The result was a 70% reduction in security incidents within the first year, thanks to improved detection and response times.
Case Study 2: E-commerce Platform
An e-commerce platform integrated machine learning to identify fraudulent transactions. The system not only flagged potentially malicious activities in real-time but also successfully reduced fraudulent transactions by 50%, safeguarding both the consumer and the brand's reputation.
Best Practices for Integrating Malware Machine Learning in Your Business
To harness the full potential of malware machine learning, businesses should consider the following best practices:
1. Invest in Quality Data
High-quality, diverse datasets are critical for training effective machine learning models. Businesses should prioritize collecting extensive data that represents a variety of potential threats.
2. Partner with Experts
Engaging with cybersecurity professionals who specialize in machine learning can facilitate more effective implementation and monitoring strategies tailored to your needs.
3. Continuously Update Models
The cybersecurity landscape is constantly evolving. Regularly updating machine learning models with new data and threat intelligence is essential to maintaining effectiveness against emerging threats.
4. Cultivate a Security-First Culture
Educating staff about cybersecurity risks and best practices ensures a holistic approach to security, which complements technological solutions.
The Future of Malware Machine Learning
The future of malware machine learning is promising. As technology advances, we can expect to see:
- Greater Collaboration: Increased collaboration between machine learning systems will lead to collective intelligence, enhancing detection accuracy across industries.
- Integration with Other Technologies: The combination of machine learning with blockchain and IoT technologies will create more comprehensive security architectures.
- Focus on User Privacy: As privacy regulations tighten, machine learning models will likely evolve to comply with these laws while still providing effective threat detection.
Conclusion
In conclusion, the integration of malware machine learning into IT services and security systems marks a significant advancement in the fight against cyber threats. By leveraging advanced algorithms and data analysis techniques, businesses can protect their assets, ensure operational resilience, and secure customer trust. For those looking to bolster their security measures, investing in machine learning solutions is not just a trend; it is a necessity for future-proofing against the inevitable—and ever-evolving—threat landscape.